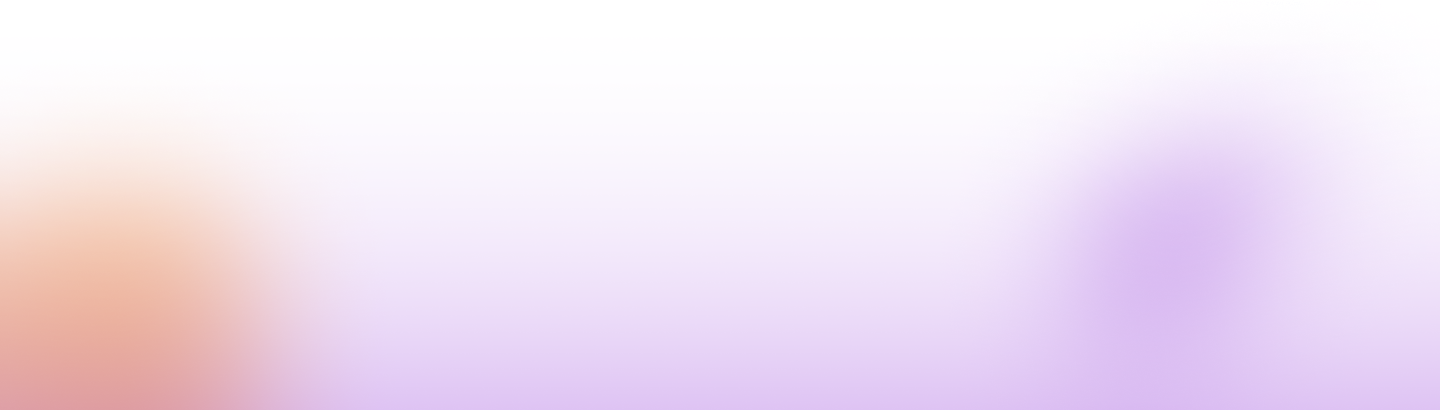
Run AI
Visit- Introduction:
Optimize GPU usage and streamline AI workflows at scale.
- Added on:
Dec 31 2024
- Company:
Runai Labs Ltd.
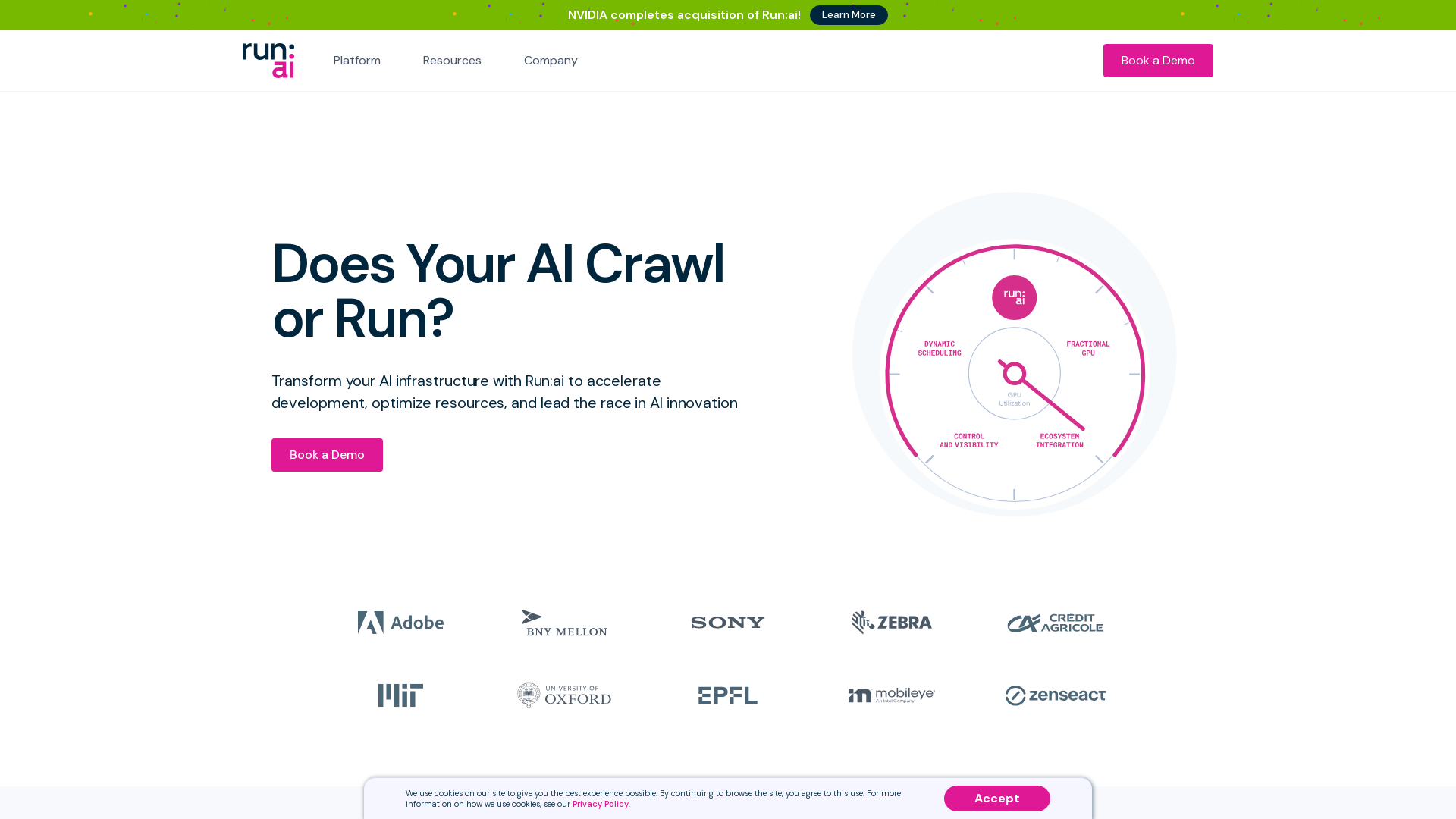
Introduction to Run:ai
Run:ai is a comprehensive platform designed to optimize GPU usage and manage AI and machine learning workflows efficiently. It enables organizations to scale their AI operations across cloud, on-premises, and hybrid environments. The platform offers a suite of tools for workload orchestration, multi-GPU management, and deep learning acceleration, helping data science, MLOps, and DevOps teams to enhance productivity and achieve faster results in AI model development and deployment.
Main Functions of Run:ai
GPU Optimization
Example
Using Run:ai, data scientists can efficiently manage multi-GPU environments for complex AI model training.
Scenario
Maximize the utilization of GPU resources across AI workloads to speed up model training and reduce idle times.
AI Workflow Automation
Example
Run:ai integrates with Kubernetes to automate the management of AI workflows, scheduling jobs and ensuring smooth execution.
Scenario
Streamline the deployment and orchestration of machine learning pipelines, from data preparation to model deployment, using automated workflows.
Hyperparameter Tuning
Example
Run:ai allows teams to fine-tune hyperparameters across multiple models simultaneously, improving the accuracy and efficiency of AI models.
Scenario
Optimize AI model performance by automating the hyperparameter tuning process, ensuring the best parameters are selected for each task.
Ideal Users of Run:ai
Data Scientists & ML Engineers
Data scientists and machine learning engineers who need to manage large-scale AI model training and resource allocation effectively.
MLOps Teams
MLOps teams looking to automate and scale machine learning workflows across hybrid cloud environments, ensuring efficient use of compute resources.
DevOps Teams
DevOps teams seeking to optimize infrastructure resources for GPU-heavy AI workloads, ensuring that resources are utilized effectively and jobs are efficiently scheduled.
Visit Over Time
- Monthly Visits248,013
- Avg.Visit Duration00:03:51
- Page per Visit5.22
- Bounce Rate46.22%
Geography
- United States42.47%
- Switzerland5.81%
- India5.37%
- United Kingdom5.31%
- Germany2.44%
Traffic Sources
Steps to Use Run:ai
- 1
Step 1: Sign Up & Choose Deployment
Sign up for Run:ai and create your account. Choose your preferred deployment type (cloud, on-premises, or hybrid).
- 2
Step 2: Configure AI Infrastructure
Set up your GPU resources and integrate your existing AI/ML tools and frameworks like TensorFlow or PyTorch.
- 3
Step 3: Optimize & Scale AI Workflows
Start running and optimizing your AI workflows. Use Run:ai’s tools for workload orchestration, hyperparameter tuning, and GPU optimization.
Frequently Asked Questions
Run AI Pricing
For the latest pricing, please visit this link:https://www.run.ai/pricing
Starter
$0/month
Basic access to GPU optimization tools
Limited access to AI/ML workflow management
Single GPU support for small-scale projects
Professional
$199/month or $2,388/year
Full access to GPU optimization and management tools
Support for multi-GPU environments
Advanced AI/ML workflow management features
Integration with Kubernetes and Slurm
Priority customer support
Enterprise
$499/month or $5,988/year
All features of the Professional tier
Customizable deployment options (cloud, on-premises, hybrid)
Advanced security features and compliance support
Dedicated account manager
Scalable resources for large organizations